-
-
Notifications
You must be signed in to change notification settings - Fork 332
/
60-minute-blitz.jl
447 lines (317 loc) · 13.9 KB
/
60-minute-blitz.jl
1
2
3
4
5
6
7
8
9
10
11
12
13
14
15
16
17
18
19
20
21
22
23
24
25
26
27
28
29
30
31
32
33
34
35
36
37
38
39
40
41
42
43
44
45
46
47
48
49
50
51
52
53
54
55
56
57
58
59
60
61
62
63
64
65
66
67
68
69
70
71
72
73
74
75
76
77
78
79
80
81
82
83
84
85
86
87
88
89
90
91
92
93
94
95
96
97
98
99
100
101
102
103
104
105
106
107
108
109
110
111
112
113
114
115
116
117
118
119
120
121
122
123
124
125
126
127
128
129
130
131
132
133
134
135
136
137
138
139
140
141
142
143
144
145
146
147
148
149
150
151
152
153
154
155
156
157
158
159
160
161
162
163
164
165
166
167
168
169
170
171
172
173
174
175
176
177
178
179
180
181
182
183
184
185
186
187
188
189
190
191
192
193
194
195
196
197
198
199
200
201
202
203
204
205
206
207
208
209
210
211
212
213
214
215
216
217
218
219
220
221
222
223
224
225
226
227
228
229
230
231
232
233
234
235
236
237
238
239
240
241
242
243
244
245
246
247
248
249
250
251
252
253
254
255
256
257
258
259
260
261
262
263
264
265
266
267
268
269
270
271
272
273
274
275
276
277
278
279
280
281
282
283
284
285
286
287
288
289
290
291
292
293
294
295
296
297
298
299
300
301
302
303
304
305
306
307
308
309
310
311
312
313
314
315
316
317
318
319
320
321
322
323
324
325
326
327
328
329
330
331
332
333
334
335
336
337
338
339
340
341
342
343
344
345
346
347
348
349
350
351
352
353
354
355
356
357
358
359
360
361
362
363
364
365
366
367
368
369
370
371
372
373
374
375
376
377
378
379
380
381
382
383
384
385
386
387
388
389
390
391
392
393
394
395
396
397
398
399
400
401
402
403
404
405
406
407
408
409
410
411
412
413
414
415
416
417
418
419
420
421
422
423
424
425
426
427
428
429
430
431
432
433
434
435
436
437
438
439
440
441
442
443
444
445
446
447
# Deep Learning with Flux: A 60 Minute Blitz
# =====================
# This is a quick intro to [Flux](https://github.com/FluxML/Flux.jl) loosely
# based on [PyTorch's
# tutorial](https://pytorch.org/tutorials/beginner/deep_learning_60min_blitz.html).
# It introduces basic Julia programming, as well Zygote, a source-to-source
# automatic differentiation (AD) framework in Julia.
# We'll use these tools to build a very simple neural network.
# Arrays
# -------
# The starting point for all of our models is the `Array` (sometimes referred to
# as a `Tensor` in other frameworks). This is really just a list of numbers,
# which might be arranged into a shape like a square. Let's write down an array
# with three elements.
x = [1, 2, 3]
# Here's a matrix – a square array with four elements.
x = [1 2; 3 4]
# We often work with arrays of thousands of elements, and don't usually write
# them down by hand. Here's how we can create an array of 5×3 = 15 elements,
# each a random number from zero to one.
x = rand(5, 3)
# There's a few functions like this; try replacing `rand` with `ones`, `zeros`,
# or `randn` to see what they do.
# By default, Julia works stores numbers is a high-precision format called
# `Float64`. In ML we often don't need all those digits, and can ask Julia to
# work with `Float32` instead. We can even ask for more digits using `BigFloat`.
x = rand(BigFloat, 5, 3)
#-
x = rand(Float32, 5, 3)
# We can ask the array how many elements it has.
length(x)
# Or, more specifically, what size it has.
size(x)
# We sometimes want to see some elements of the array on their own.
x
#-
x[2, 3]
# This means get the second row and the third column. We can also get every row
# of the third column.
x[:, 3]
# We can add arrays, and subtract them, which adds or subtracts each element of
# the array.
x + x
#-
x - x
# Julia supports a feature called *broadcasting*, using the `.` syntax. This
# tiles small arrays (or single numbers) to fill bigger ones.
x .+ 1
# We can see Julia tile the column vector `1:5` across all rows of the larger
# array.
zeros(5,5) .+ (1:5)
# The x' syntax is used to transpose a column `1:5` into an equivalent row, and
# Julia will tile that across columns.
zeros(5,5) .+ (1:5)'
# We can use this to make a times table.
(1:5) .* (1:5)'
# Finally, and importantly for machine learning, we can conveniently do things like
# matrix multiply.
W = randn(5, 10)
x = rand(10)
W * x
# Julia's arrays are very powerful, and you can learn more about what they can
# do [here](https://docs.julialang.org/en/v1/manual/arrays/).
# ### CUDA Arrays
# CUDA functionality is provided separately by the [CUDA
# package](https://github.com/JuliaGPU/CUDA.jl). If you have a GPU and CUDA
# available, you can run `] add CUDA` in a REPL or IJulia to get it.
# Once CUDA is loaded you can move any array to the GPU with the `cu`
# function, and it supports all of the above operations with the same syntax.
## using CUDA
## x = cu(rand(5, 3))
# Automatic Differentiation
# -------------------------
# You probably learned to take derivatives in school. We start with a simple
# mathematical function like
f(x) = 3x^2 + 2x + 1
f(5)
# In simple cases it's pretty easy to work out the gradient by hand – here it's
# `6x+2`. But it's much easier to make Flux do the work for us!
using Flux: gradient
df(x) = gradient(f, x)[1]
df(5)
# You can try this with a few different inputs to make sure it's really the same
# as `6x+2`. We can even do this multiple times (but the second derivative is a
# fairly boring `6`).
ddf(x) = gradient(df, x)[1]
ddf(5)
# Flux's AD can handle any Julia code you throw at it, including loops,
# recursion and custom layers, so long as the mathematical functions you call
# are differentiable. For example, we can differentiate a Taylor approximation
# to the `sin` function.
mysin(x) = sum((-1)^k*x^(1+2k)/factorial(1+2k) for k in 0:5)
x = 0.5
mysin(x), gradient(mysin, x)
#-
sin(x), cos(x)
# You can see that the derivative we calculated is very close to `cos(x)`, as we
# expect.
# This gets more interesting when we consider functions that take *arrays* as
# inputs, rather than just a single number. For example, here's a function that
# takes a matrix and two vectors (the definition itself is arbitrary)
myloss(W, b, x) = sum(W * x .+ b)
W = randn(3, 5)
b = zeros(3)
x = rand(5)
gradient(myloss, W, b, x)
# Now we get gradients for each of the inputs `W`, `b` and `x`, which will come
# in handy when we want to train models.
# Because ML models can contain hundreds of parameters, Flux provides a slightly
# different way of writing `gradient`. We instead mark arrays with `param` to
# indicate that we want their derivatives. `W` and `b` represent the weight and
# bias respectively.
using Flux: params
W = randn(3, 5)
b = zeros(3)
x = rand(5)
y(x) = sum(W * x .+ b)
grads = gradient(()->y(x), params([W, b]))
grads[W], grads[b]
# We can now grab the gradients of `W` and `b` directly from those parameters.
# This comes in handy when working with *layers*. A layer is just a handy
# container for some parameters. For example, `Dense` does a linear transform
# for you.
using Flux
m = Dense(10, 5)
# We can easily get the parameters of any layer or model with params with
# `params`.
params(m)
# This makes it very easy to calculate the gradient for all
# parameters in a network, even if it has many parameters.
x = rand(Float32, 10)
m = Chain(Dense(10, 5, relu), Dense(5, 2), softmax)
l(x) = Flux.Losses.crossentropy(m(x), [0.5, 0.5])
grads = gradient(params(m)) do
l(x)
end
for p in params(m)
println(grads[p])
end
# You don't have to use layers, but they can be convenient for many simple kinds
# of models and fast iteration.
# The next step is to update our weights and perform optimisation. As you might be
# familiar, *Gradient Descent* is a simple algorithm that takes the weights and steps
# using a learning rate and the gradients. `weights = weights - learning_rate * gradient`
# (note that `Flux.Optimise.update!(x, x̄)` already updates with the negative of x̄`).
using Flux.Optimise: update!, Descent
η = 0.1
for p in params(m)
update!(p, η * grads[p])
end
# While this is a valid way of updating our weights, it can get more complicated as the
# algorithms we use get more involved.
# Flux comes with a bunch of pre-defined optimisers and makes writing our own really simple.
# We just give it the learning rate η
opt = Descent(0.01)
# `Training` a network reduces down to iterating on a dataset multiple times, performing these
# steps in order. Just for a quick implementation, let’s train a network that learns to predict
# `0.5` for every input of 10 floats. `Flux` defines the `train!` function to do it for us.
data, labels = rand(10, 100), fill(0.5, 2, 100)
loss(x, y) = Flux.Losses.crossentropy(m(x), y)
Flux.train!(loss, params(m), [(data,labels)], opt)
# You don't have to use `train!`. In cases where arbitrary logic might be better suited,
# you could open up this training loop like so:
# ```julia
# for d in training_set # assuming d looks like (data, labels)
# # our super logic
# gs = gradient(params(m)) do #m is our model
# l = loss(d...)
# end
# update!(opt, params(m), gs)
# end
# ```
# Training a Classifier
# ---------------------
# Getting a real classifier to work might help cement the workflow a bit more.
# [CIFAR10](url) is a dataset of 50k tiny training images split into 10 classes.
# We will do the following steps in order:
# * Load CIFAR10 training and test datasets
# * Define a Convolution Neural Network
# * Define a loss function
# * Train the network on the training data
# * Test the network on the test data
# Loading the Dataset
# [Metalhead.jl](https://github.com/FluxML/Metalhead.jl) is an excellent package
# that has a number of predefined and pretrained computer vision models.
# It also has a number of dataloaders that come in handy to load datasets.
using Statistics
using Flux, Flux.Optimise
using MLDatasets: CIFAR10
using Images.ImageCore
using Flux: onehotbatch, onecold
using Base.Iterators: partition
using CUDA
# The image will give us an idea of what we are dealing with.
# 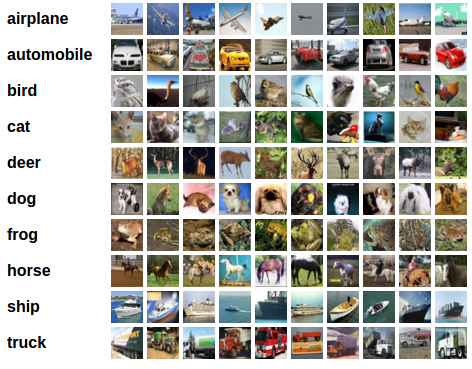
train_x, train_y = CIFAR10(:train)[:]
labels = onehotbatch(train_y, 0:9)
#The train_x contains 50000 images converted to 32 X 32 X 3 arrays with the third
# dimension being the 3 channels (R,G,B). Let's take a look at a random image from
# the train_x. For this, we need to permute the dimensions to 3 X 32 X 32 and use
# `colorview` to convert it back to an image.
# Let's take a look at a random image from the dataset
using Plots
image(x) = colorview(RGB, permutedims(x, (3, 2, 1)))
plot(image(train_x[:,:,:,rand(1:end)]))
# The images are simply 32 X 32 matrices of numbers in 3 channels (R,G,B). We can now
# arrange them in batches of say, 1000 and keep a validation set to track our progress.
# This process is called minibatch learning, which is a popular method of training
# large neural networks. Rather that sending the entire dataset at once, we break it
# down into smaller chunks (called minibatches) that are typically chosen at random,
# and train only on them. It is shown to help with escaping
# [saddle points](https://en.wikipedia.org/wiki/Saddle_point).
# The first 49k images (in batches of 1000) will be our training set, and the rest is
# for validation. `partition` handily breaks down the set we give it in consecutive parts
# (1000 in this case).
train = ([(train_x[:,:,:,i], labels[:,i]) for i in partition(1:49000, 1000)]) |> gpu
valset = 49001:50000
valX = train_x[:,:,:,valset] |> gpu
valY = labels[:, valset] |> gpu
# ## Defining the Classifier
# --------------------------
# Now we can define our Convolutional Neural Network (CNN).
# A convolutional neural network is one which defines a kernel and slides it across a matrix
# to create an intermediate representation to extract features from. It creates higher order
# features as it goes into deeper layers, making it suitable for images, where the structure of
# the subject is what will help us determine which class it belongs to.
m = Chain(
Conv((5,5), 3=>16, relu),
MaxPool((2,2)),
Conv((5,5), 16=>8, relu),
MaxPool((2,2)),
x -> reshape(x, :, size(x, 4)),
Dense(200, 120),
Dense(120, 84),
Dense(84, 10),
softmax) |> gpu
#-
# We will use a crossentropy loss and the Momentum optimiser here. Crossentropy will be a
# good option when it comes to working with multiple independent classes. Momentum smooths out
# the noisy gradients and helps towards a smooth convergence. Gradually lowering the
# learning rate along with momentum helps to maintain a bit of adaptivity in our optimisation,
# preventing us from overshooting our desired destination.
#-
using Flux: crossentropy, Momentum
loss(x, y) = sum(crossentropy(m(x), y))
opt = Momentum(0.01)
# We can start writing our train loop where we will keep track of some basic accuracy
# numbers about our model. We can define an `accuracy` function for it like so.
accuracy(x, y) = mean(onecold(m(x), 0:9) .== onecold(y, 0:9))
# ## Training
# -----------
# Training is where we do a bunch of the interesting operations we defined earlier,
# and see what our net is capable of. We will loop over the dataset 10 times and
# feed the inputs to the neural network and optimise.
epochs = 10
for epoch = 1:epochs
for d in train
gs = gradient(params(m)) do
l = loss(d...)
end
update!(opt, params(m), gs)
end
@show accuracy(valX, valY)
end
# Seeing our training routine unfold gives us an idea of how the network learnt the
# This is not bad for a small hand-written network, trained for a limited time.
# Training on a GPU
# -----------------
# The `gpu` functions you see sprinkled through this bit of the code tell Flux to move
# these entities to an available GPU, and subsequently train on it. No extra faffing
# about required! The same bit of code would work on any hardware with some small
# annotations like you saw here.
# ## Testing the Network
# ----------------------
# We have trained the network for 10 passes over the training dataset. But we need to
# check if the network has learnt anything at all.
# We will check this by predicting the class label that the neural network outputs, and
# checking it against the ground-truth. If the prediction is correct, we add the sample
# to the list of correct predictions. This will be done on a yet unseen section of data.
# Okay, first step. Let us perform the exact same preprocessing on this set, as we did
# on our training set.
test_x, test_y = CIFAR10(:test)[:]
test_labels = onehotbatch(test_y, 0:9)
test = gpu.([(test_x[:,:,:,i], test_labels[:,i]) for i in partition(1:10000, 1000)])
# Next, display an image from the test set.
plot(image(test_x[:,:,:,rand(1:end)]))
# The outputs are energies for the 10 classes. Higher the energy for a class, the more the
# network thinks that the image is of the particular class. Every column corresponds to the
# output of one image, with the 10 floats in the column being the energies.
# Let's see how the model fared.
ids = rand(1:10000, 5)
rand_test = test_x[:,:,:,ids] |> gpu
rand_truth = test_y[ids]
m(rand_test)
# This looks similar to how we would expect the results to be. At this point, it's a good
# idea to see how our net actually performs on new data, that we have prepared.
accuracy(test[1]...)
# This is much better than random chance set at 10% (since we only have 10 classes), and
# not bad at all for a small hand written network like ours.
# Let's take a look at how the net performed on all the classes performed individually.
class_correct = zeros(10)
class_total = zeros(10)
for i in 1:10
preds = m(test[i][1])
lab = test[i][2]
for j = 1:1000
pred_class = findmax(preds[:, j])[2]
actual_class = findmax(lab[:, j])[2]
if pred_class == actual_class
class_correct[pred_class] += 1
end
class_total[actual_class] += 1
end
end
class_correct ./ class_total
# The spread seems pretty good, with certain classes performing significantly better than the others.
# Why should that be?